TIMELINE
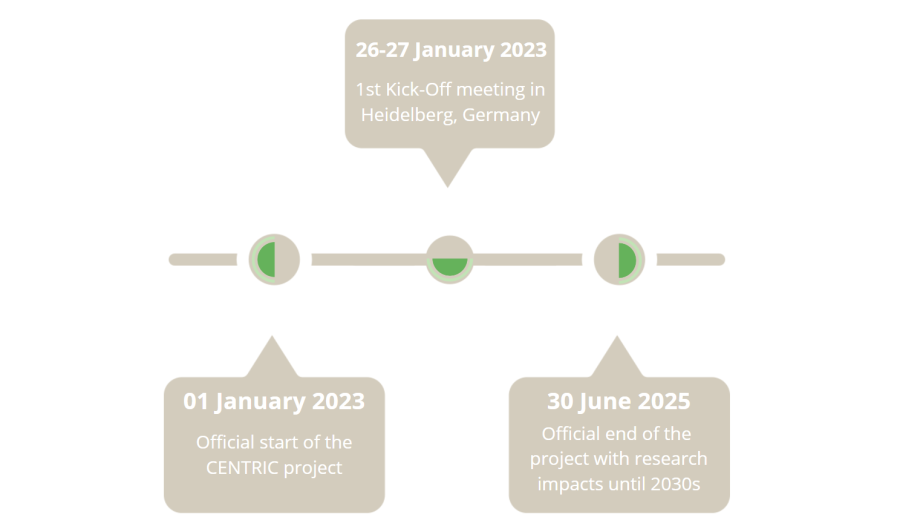
CHALLENGES
The transformation of current mobile communication networks towards a user-centric paradigm has increasingly concentrated the attention of academia and industry over the last decade. At the onset of the 5G standardization process, it was predicted that designing a user-centric architecture would be one of the main disruptive goals of 5G. Since then, significant progress has been made towards the idea of user-centric communications at the network layer. When it comes to the air interface, however, progress has been much slower. The current 5G air interface follows a service-centric approach, rather than a user-centric one: The physical layer design, along with the access protocols and radio-resource management are designed such that co-existence of multiple wireless services is possible. These services have been broadly categorized into three distinct classes: eMBB, mMTC, and ultra-reliable low-latency communications (URLLC). Using OFDM as a basis, the 5G air interface permits a myriad of configurations that allow for a given cell or group of cells to adapt the air interface to the most prevalent services that the respective users require. Such an approach, however, is hardly scalable to the expected increase in the number of services and applications that will be provided by the networks of the future, as the complexity of defining, standardising, and deploying configurations optimized for all possible users’ requirements is practically unmanageable.
Addressing these challenges, which are listed next, is the main goal of CENTRIC.
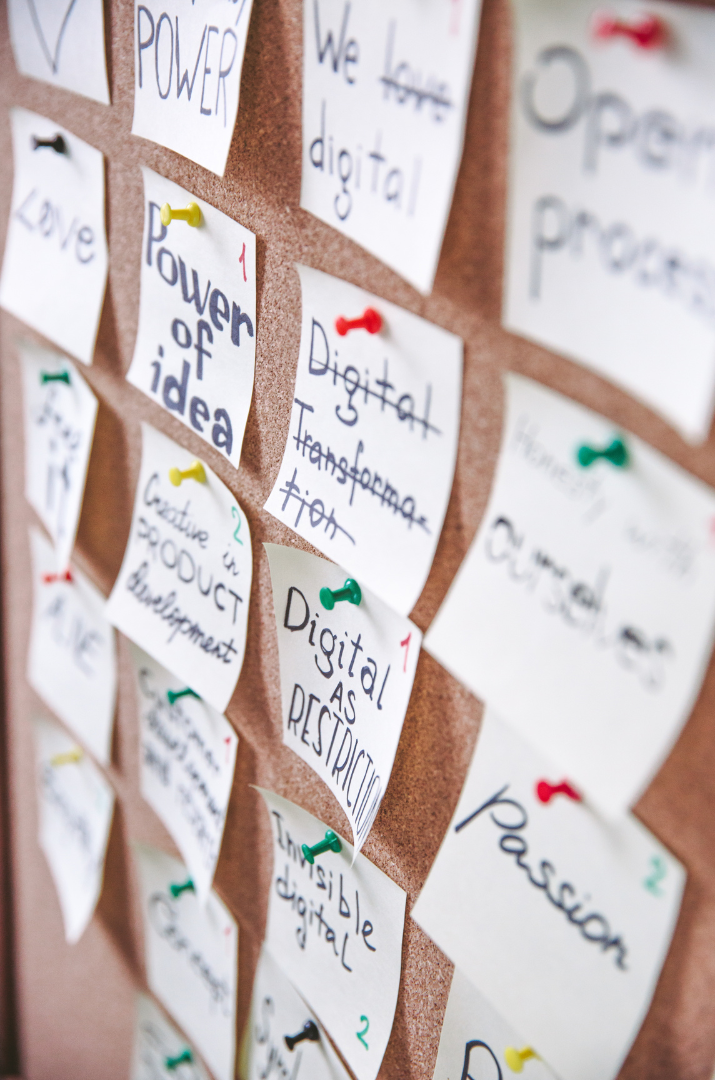
Traditionally, PHY procedures have been designed following a layered approach, in which different tasks and functionalities are optimized with respect to their own performance criteria. In the pioneering work, a new paradigm was proposed: the use of deep neural networks as autoencoders representing the full PHY chain allows for jointly designing transmit and receive processing with a joint, end-to-end (E2E) objective function. Despite significant efforts over the last five years, it is still unclear whether such an approach can lead to practical, energy-efficient, and high-performing waveforms, modulation and code designs within a modular architecture that builds on –rather than ignoring—the model-based engineering and theoretical insights developed over the last seventy years.
Among the positive results in this direction are AI methods for MIMO processing. Over the past couple of generations, MIMO communications have arguably been the leading technology for spectral efficiency improvements in mobile networks and will continue to be of paramount importance in 6G. While high-performing model-based processing methods for MIMO communications exist, their high complexity is a bottleneck for the current trend of increasing number of antenna elements at transceivers. AI-aided methods can help alleviate this complexity burden, especially at high frequencies (mmWave, sub-THz, and THz ranges), due to their ability to fuse information from multiple sensors such as image-based sensors, light detection and ranging (LIDAR), radar, and RF.
While the application of AI methods to the PHY has given rise to much research over the last few years, its impact on the MAC layer has been much less investigated. Recently, however, it has been shown that the framework of MARL can succeed at engineering MAC protocols from scratch through a goal-driven approach. Since these results are obtained using simplified signal models, there is a need to explore their scalability to more realistic scenarios and complex tasks.
Coverage and capacity used to be the two most important KPIs for mobile network operators. The United Nations (UN) Sustainable Development Goals (SDGs) and the recent energy crisis have changed this by making energy efficiency (typically measured in bit/Joule) an important metric with which to evaluate communication systems. For this reason, the cross-layer solutions to be developed by CENTRIC will treat energy efficiency and power consumption as one main optimization target, on par with the traditional KPIs of coverage, bitrate and latency. At layer-1 (L1), AI techniques must not just learn a new spectrally-efficient waveform. It must also be energy-efficient. At L2, multiple-access protocols must minimize wasted transmissions and minimize collision rates, while also controlling the impact of this on latency. At the RRM layer, AI algorithms can leverage an abundance of network-wide data to provide energy-efficient solutions in various problems (e.g., cell switch-off for energy savings that satisfy minimum QoS requirements). In general, black-box optimization techniques such as Bayesian optimization, as well as learn-from-experience AI techniques such as reinforcement learning have the potential to leverage myriads of data to provide effective solutions that remain obscure to human intuitions across all layers in the stack.
The problem of radio resource allocation in cell-free massive MIMO networks with EMF exposure constraints is a multi-objective one. Beams in a cell-free network must be steered dynamically to guarantee the user QoE, and this must be done while respecting EMF limits. Once again, such a scenario can produce abundant data that AI techniques can leverage better than humans. However, these data-hungry methods have the downside of requiring excessive power. Networkwide, techniques such as federated learning, or ML-model caching can contribute to reducing the complexity and energy footprint of distributed learning methods.
A crucial challenge in the path towards an AI-native, user-centric air interface design is the management of the data and computations necessary to train and maintain the repository of AI models. While ideally AI models should be trained (and re-trained) using data gathered in real-life networks, the collection of such data in all deployment scenarios, for all applications, and for all user types, involves excessive overhead and energy expenditure that render this option infeasible. Instead, virtual models of the environment –in the form of DT—can be used to alleviate the burden of real-world data demands. In addition, such virtual environments can be used for monitoring the performance of already trained AI models against changes in the environment, as well as user demands. While development of DTs is expected to boom in the coming years, there is a need for sound theoretical frameworks and efficient algorithms that can leverage interaction between virtual and real environments to train and continuously adapt AI models with a realistic amount of data, computation, and energy consumption.
To enable the CENTRIC vision, it is crucial that AI training and inference computations be able to run on fast and energy-efficient hardware platforms at the edge and at mobile devices. To this end, AI algorithms should be designed in a way that suits the characteristics of the computational platforms used to run them. Thus, there is a need for co-designing the hardware platforms and AI algorithms together, to yield practically feasible AI-based air-interface components. The interplay between algorithms and hardware platforms should be addressed not only via currently dominant deep learning models for GPU computations, but also by exploring novel computing paradigms such as neuromorphic computing as more efficient alternatives to state-of-the-art solutions.
The last main challenge on the way towards an AI-enabled, user-centric air interface is the need for validating and testing the performance of AI-aided protocols. The difficulty arises from the inherent lack of transparency and interpretability of AI models, which often work as black boxes whose inner workings are not specified by the designer. If AI is to be a central component of 6G networks, there is a need for dependable, robust and insightful testing procedures that can be used to verify that the different network components.