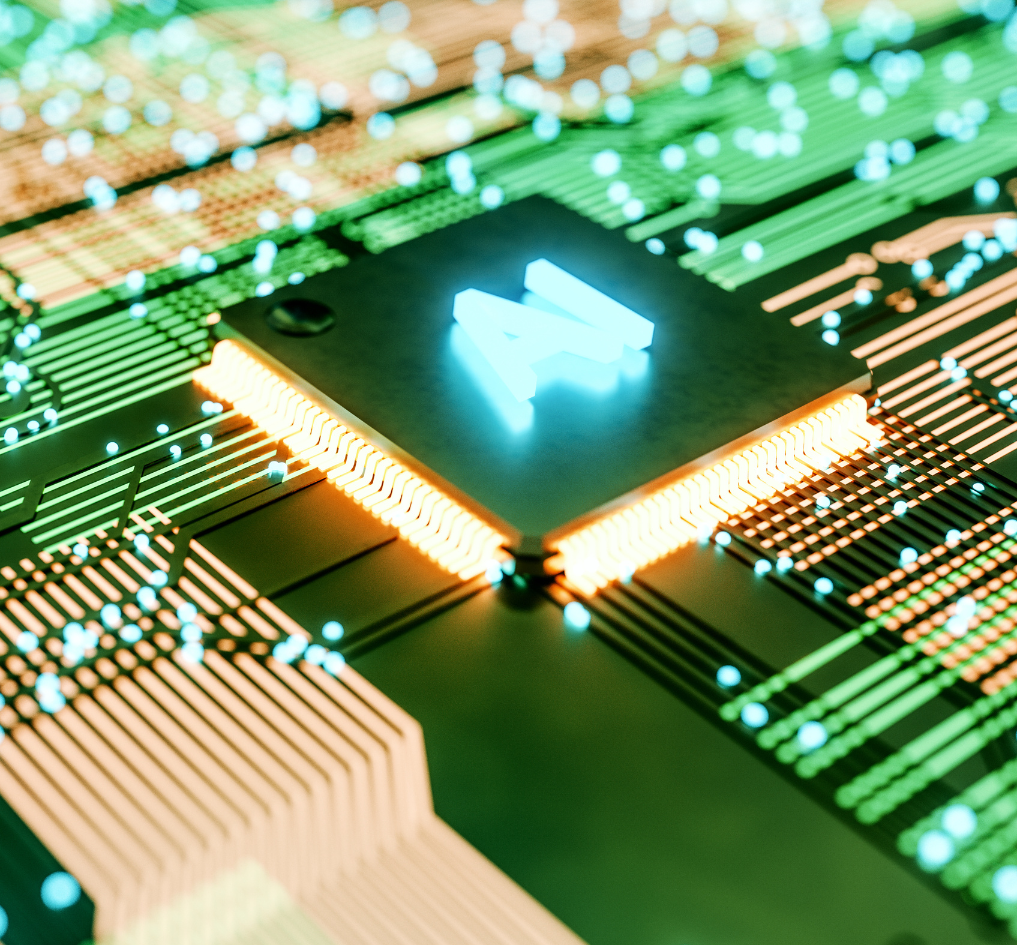
Use cases
CENTRIC develops and tests the theories, methods, and technologies necessary to bring the vision of an AI-enabled user-centric and energy-efficient air interface to the level of maturity that make it feasible in future 6G systems. This entails the methods and processes necessary to automatically produce a connectivity solution that fulfils the needs of a particular user in a specific environment, as well as to dynamically adapt to changes in the environment and/or the user’s context.
The increase in the number of services and applications is a very real phenomenon and not just a telecoms-industry cliche. Before the COVID19 pandemic and the work-from-home revolution, few telecom experts would have voted for screen sharing as a relevant traffic type. Today, it is one of the most important telecom services, but the underlying network has not been adapted to support it. New services and applications emerge all the time and we need a new methodology to customize our networks to support them.
Objectives
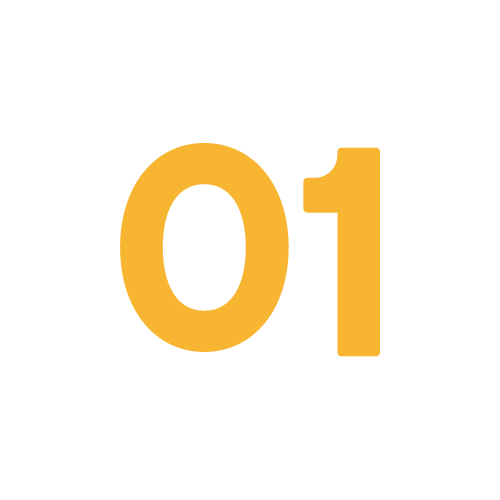
Objective 1 – To develop AI methods for the discovery of novel and efficient waveforms
At the physical layer, CENTRIC will develop ML techniques that enable the discovery and optimization of tailor-made waveforms. These target the communication needs of the user while accounting for the hardware limitations of the involved RF and computing hardware, as well as for the propagation environment in which they will be deployed.
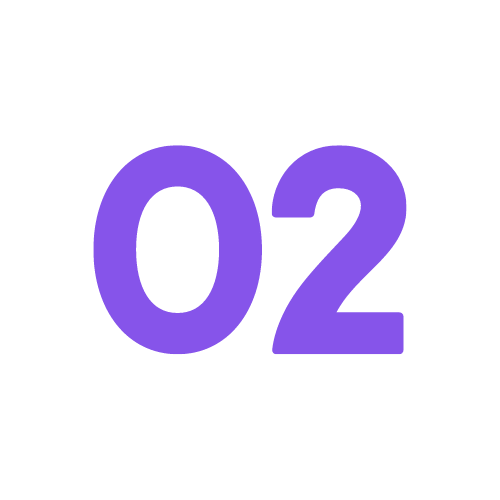
Objective 2 – To develop AI methods for the discovery of novel and efficient transceivers
CENTRIC will design efficient AI-based transceivers for two particularly challenging use-cases: large-scale extreme massive multiple-input multiple-output (MIMO) deployments and beam-based communications in millimetre-wave (mmWave) spectrum. On the former, we will focus on optimizing multi-user detection performance and devising efficient channel state information acquisition methods, while environment sensing techniques towards the improvement of beam prediction and management operations will devised on the latter.
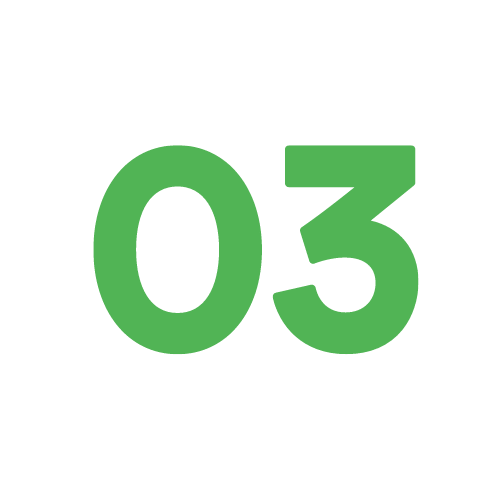
Objective 3 – To develop AI methods for the discovery of customized lightweight communication protocols
This objective will be approached via learning-to-communicate (L2C) techniques based on multi-agent reinforcement learning (MARL), semantic communications, and edge control/caching. Preliminary research by CENTRIC partners (see [2]) has demonstrated that the automated generation of communication protocols in vertical markets is realistically achievable within CENTRIC’s lifetime.
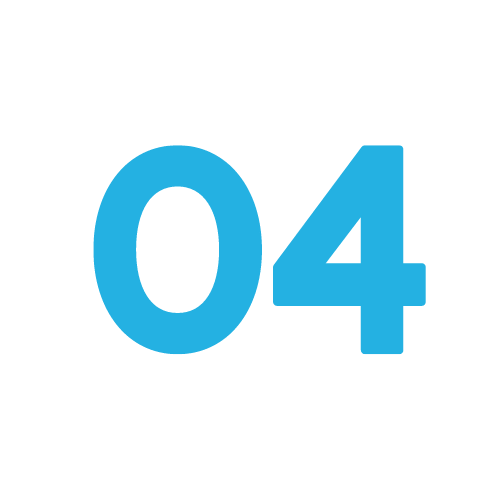
Objective 4 – To introduce novel end-to-end hardware co-design solutions for energy-efficient AI-native transceivers
AI-AI algorithms and protocols must run in dedicated computing hardware that is optimized for AI inference and that accounts for the resources available at the different network elements. The feasibility, resource requirements, and sustainability of an AI-native system hinges on an optimized integration of algorithms and hardware platforms. To this end, CENTRIC will explore, design and validate AI accelerators that are based on both standard digital technology and emerging mixed-analog digital platforms based on non-volatile memory elements.
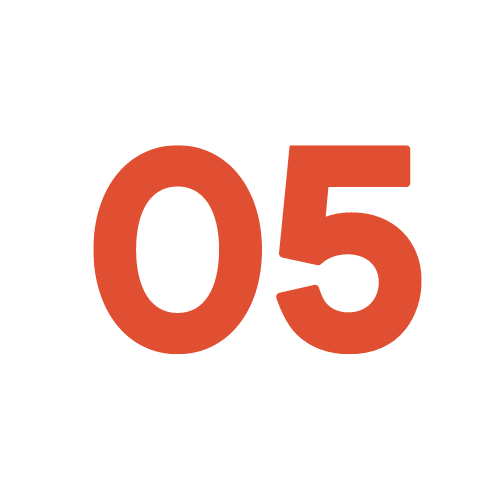
Objective 5 – To develop training and monitoring environments as enablers for AI-AI deployments
Key enablers of the AI-AI concept advocated by CENTRIC include tools that network designers and operators can deploy to train and monitor AI models. CENTRIC will adopt a general framework based on digital twins (DT), which encompasses cloud-based virtual twin agents running networked AI models of physical agent counterparts.
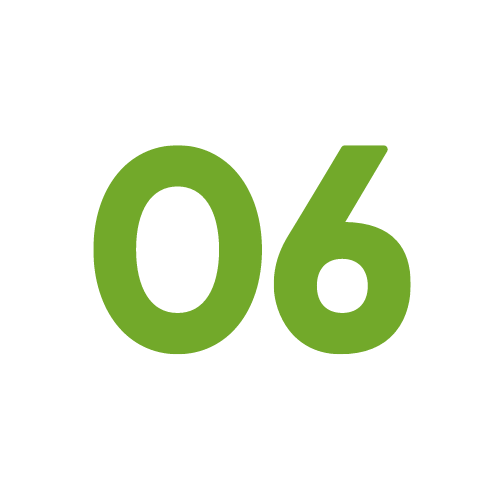
Objective 6 – To validate user-centric AI-AI solutions in a lab setting
CENTRIC will break away from the traditional standardized air interface and develop a customized user-centric one. In this case, standardized tests for a finite set of use-cases and scenarios with fixed KPI requirements will be insufficient. To fill this methodological gap, CENTRIC will define testing and benchmarking frameworks for user-centric AI-based communications.
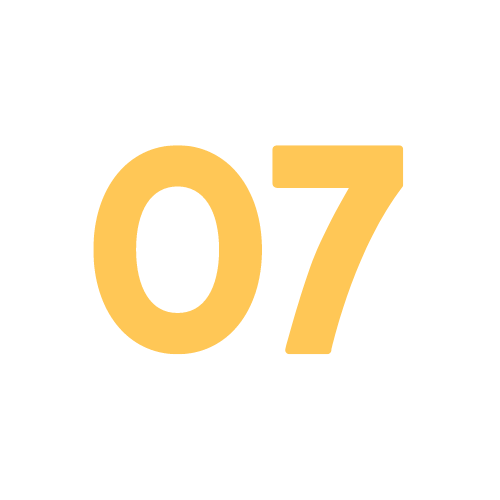
Objective 7 – To demonstrate and disseminate AI-AI concepts
CENTRIC will demonstrate novel AI-AI concepts through the development of laboratory proof-of-concept (PoC) implementations. These will validate the expected gains and shed light on the feasibility and cost of their implementation.
The outcomes of CENTRIC will target academic, industrial, and commercial communities working on the development of 6G systems. Publication of CENTRIC findings in publicly available academic literature will ensure worldwide visibility.